Quality control is a critical aspect of the manufacturing process, ensuring that products meet the desired standards of excellence before they reach consumers. Traditionally, quality control relied on human inspection, which was both time-consuming and prone to errors. However, with the advent of artificial intelligence (AI) and machine learning, manufacturers now have powerful tools at their disposal to enhance the efficiency and accuracy of quality control processes.
In this article, we will explore how AI is transforming quality control in manufacturing and provide insights into how businesses can implement AI-driven solutions to improve product quality.
1. Machine Vision and Defect Detection
One of the most significant applications of AI in quality control is machine vision. Machine vision systems use cameras and AI algorithms to inspect products for defects and anomalies. These systems can quickly and accurately identify issues that may be difficult for human inspectors to detect, such as tiny cracks, irregularities in texture, or deviations in color. Machine vision is particularly valuable in industries like automotive, electronics, and food production, where even minor defects can have serious consequences.
Machine vision systems are an important part of quality control in manufacturing. These systems are equipped with high-resolution cameras that capture detailed images of products as they move along the production line. These images are then analyzed by AI algorithms that have been trained to recognize various types of defects. The AI can flag products that deviate from the specified standards, allowing for their removal from the production line for further inspection or rework.
Machine vision is not limited to visual inspections alone. It can also employ other sensors, such as infrared or ultrasonic, to detect defects that may not be visible to the naked eye. For example, in the aerospace industry, infrared machine vision can detect heat-related issues in aircraft components, ensuring the safety and reliability of the final product.
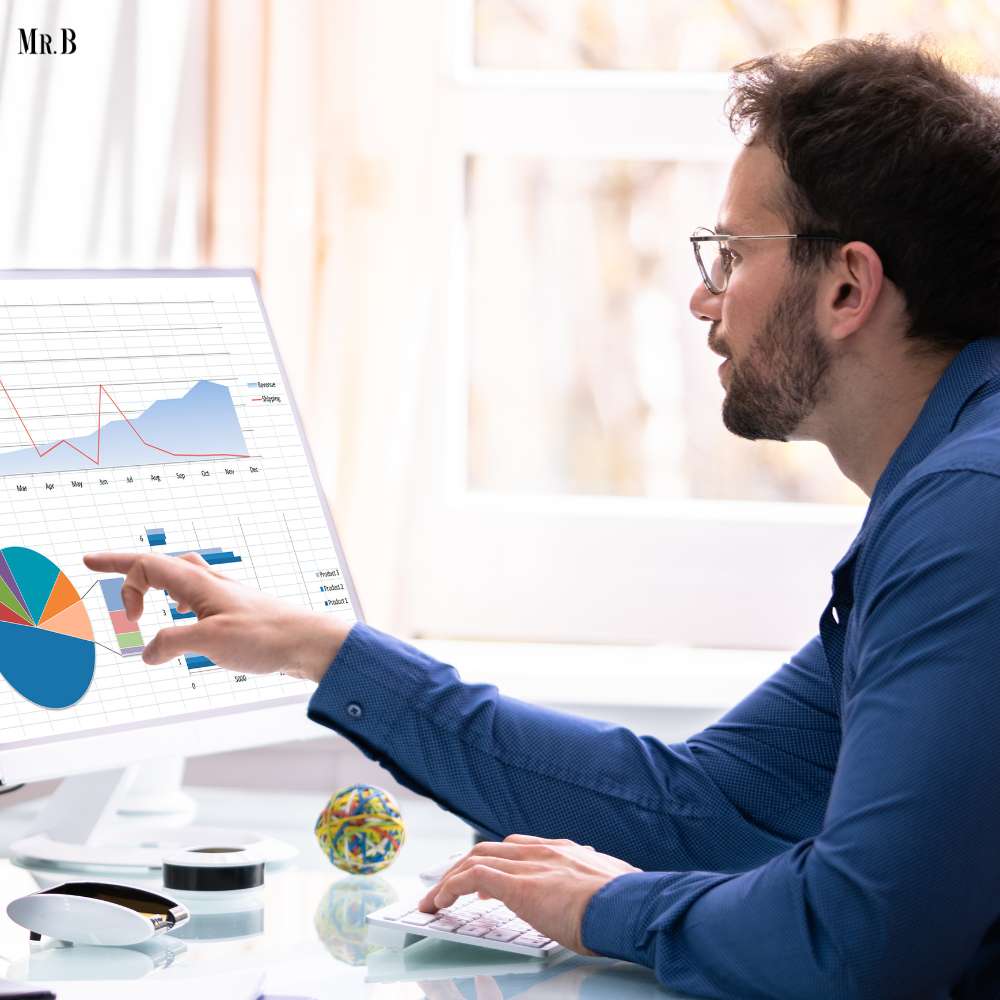
2. Predictive Maintenance
AI-powered predictive maintenance is another crucial tool for ensuring quality control in manufacturing. By analyzing data from sensors and machinery, AI can predict when equipment is likely to fail or require maintenance. This proactive approach not only reduces downtime but also helps prevent manufacturing errors caused by faulty equipment. For example, in the aerospace industry, predictive maintenance can prevent catastrophic failures by identifying issues before they compromise safety.
Predictive maintenance relies on the continuous monitoring of critical equipment using sensors that measure factors like temperature, vibration, and fluid levels. AI algorithms process this data and can detect subtle changes that may indicate early signs of equipment degradation or impending failure.
When potential issues are identified, maintenance teams can be alerted to perform necessary repairs or replacements before the equipment fails. This not only saves time and money but also ensures that quality control in manufacturing and its process remains consistent. By preventing equipment failures, manufacturers can avoid costly defects that could compromise the quality of the final product.
3. Process Optimization
When it comes to Quality Control in Manufacturing, AI can optimize various manufacturing processes to minimize defects and improve overall product quality. Machine learning algorithms can analyze production data in real-time to identify patterns and trends that may lead to defects. Manufacturers can then adjust their processes accordingly to reduce the likelihood of defects occurring. This not only enhances product quality but also increases efficiency and reduces waste.
Process optimization through AI involves several key steps:
a. Data Collection: AI systems gather data from various sources within the manufacturing process. This data includes information on machine performance, material quality, environmental conditions, and more.
b. Data Analysis: AI algorithms analyze the collected data to identify correlations, anomalies, and potential areas for improvement. These algorithms can process vast amounts of data quickly, making it possible to detect subtle patterns that may go unnoticed by human operators.
c. Process Adjustment: Based on the insights gained from data analysis, manufacturers can make real-time adjustments to their processes. These adjustments may involve changes in machine settings, material handling, or environmental conditions.
d. Continuous Monitoring: AI systems continuously monitor the adjusted processes to ensure that they are producing the desired results. If any deviations from the quality standards are detected, the system can trigger alarms or further adjustments.
e. Feedback Loop: The data generated by these adjustments is fed back into the AI system, creating a continuous feedback loop. This allows the system to learn and adapt over time, further improving process efficiency and product quality.
Process optimization through AI is not a one-time effort but an ongoing process of refinement. Manufacturers can fine-tune their processes continually to achieve the highest levels of quality and efficiency.
4. Real-time Monitoring
Real-time monitoring of processes is essential for maintaining consistent product quality control in manufacturing. AI systems can continuously monitor various parameters, such as temperature, pressure, and humidity, to ensure they remain within specified tolerances. If any parameters deviate from the acceptable range, the system can automatically trigger alarms or make adjustments to bring the process back into compliance. This level of automation and monitoring is especially valuable in industries like pharmaceuticals, where strict quality standards must be met.
Real-time monitoring is made possible through the use of sensors and IoT (Internet of Things) devices that collect data from different points in the manufacturing process. AI algorithms analyze this data in real-time, allowing for immediate responses to any deviations or anomalies.
For example, in semiconductor manufacturing, maintaining precise temperature and humidity levels is crucial to prevent defects in the final product. AI-powered monitoring systems can adjust environmental conditions as needed to ensure that the semiconductor fabrication process proceeds without interruptions or errors.
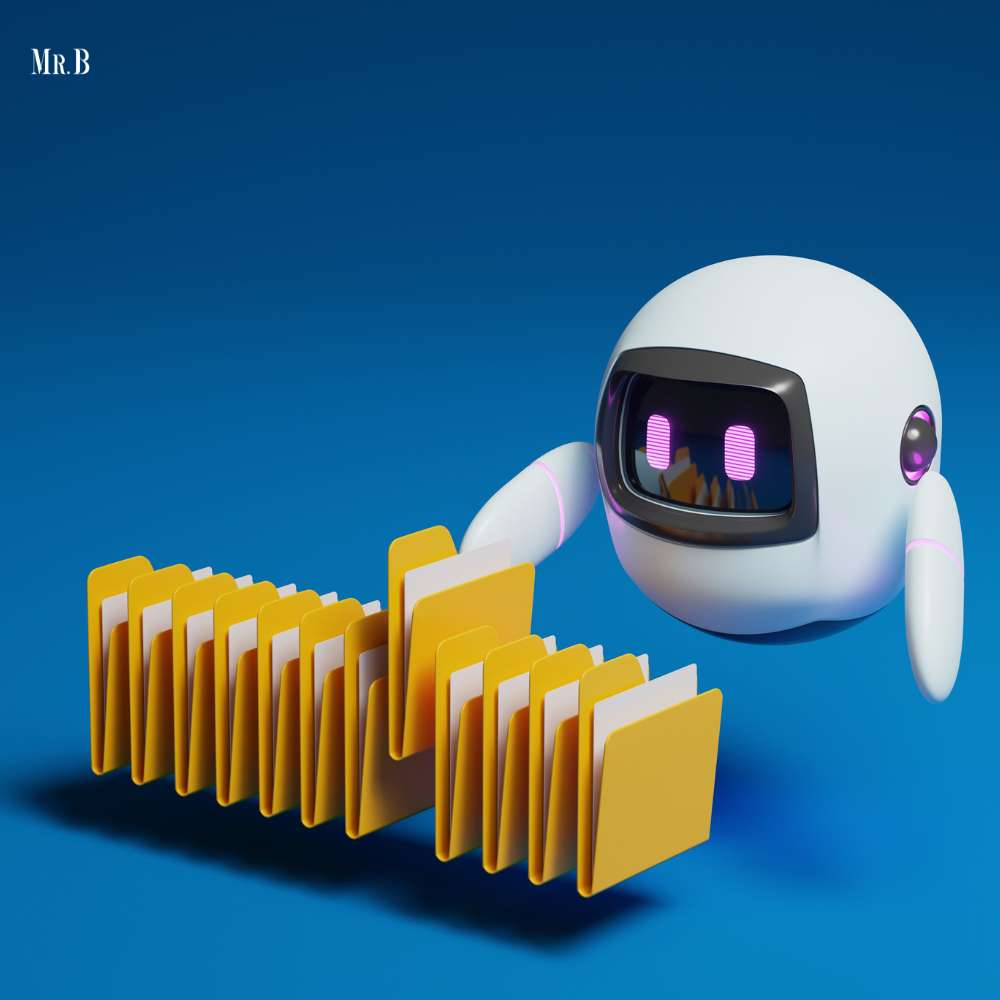
5. Quality Data Analysis
AI-driven quality control in manufacturing generates a vast amount of data that can be analyzed to gain valuable insights. Manufacturers can use this data to identify the root causes of defects and take corrective actions to prevent them in the future. By applying advanced analytics, AI can uncover hidden correlations and trends that may not be apparent through traditional analysis methods. This data-driven approach can lead to continuous improvement in product quality.
Quality data analysis involves several key steps:
a. Data Collection: As part of the quality control in manufacturing, AI systems collect data on defects, their frequency, and their characteristics. This data also includes information on the production process, materials used, and environmental conditions.
b. Root Cause Analysis: AI algorithms can perform root cause analysis to identify the underlying factors contributing to defects. For example, if a certain type of defect is consistently associated with a specific machine or a particular batch of raw materials, AI can pinpoint these correlations.
c. Statistical Analysis: Advanced statistical techniques can be applied to the data to determine the significance of various factors in relation to defects. This helps manufacturers prioritize areas for improvement.
d. Predictive Modeling: AI can develop predictive models that forecast the likelihood of defects occurring under different conditions. Manufacturers can use these models to proactively address potential issues.
e. Continuous Improvement: The insights gained from data analysis can be used to implement process improvements and preventive measures. By addressing the root causes of defects, manufacturers can achieve higher product quality and lower defect rates.
6. Supply Chain Integration
Quality control in manufacturing does not stop at the factory door. AI can also play a vital role in ensuring the quality of raw materials and components in the supply chain. By integrating AI into supply chain management, manufacturers can track the quality of incoming materials, detect potential issues early, and make informed decisions about sourcing and procurement. This proactive approach helps prevent defects from entering the manufacturing process in the first place.
Supply chain integration with AI involves the following elements:
a. Supplier Quality Assessment: AI systems can assess the quality of materials and components provided by suppliers. By analyzing data on supplier performance and material quality, manufacturers can identify reliable suppliers and reduce the risk of receiving subpar materials.
b. Quality Assurance in Transit: AI can monitor the conditions in which materials and components are transported to the manufacturing facility. For example, temperature-sensitive materials can be tracked to ensure they are not exposed to temperature extremes that could affect their quality.
c. Real-time Inventory Monitoring: AI can provide real-time visibility into inventory levels and quality. Manufacturers can use this information to optimize inventory management and ensure that only high-quality materials are used in production.
d. Early Issue Detection: AI can analyze data from the supply chain to detect potential issues before they impact production. For example, if a particular batch of materials from a supplier has a history of defects, AI can alert manufacturers to the potential risk.
e. Supplier Collaboration: AI-powered communication and collaboration tools can facilitate communication with suppliers. Manufacturers can share quality requirements and receive real-time updates on the status of materials and components.
For manufacturers integrating AI into the supply chain, quality control in manufacturing can become strict from the beginning of the production process to the final product’s delivery.
7. Customizable AI Solutions
AI-powered quality control in manufacturing solutions is highly customizable to meet the specific needs of different manufacturing industries. Whether you’re producing electronics, pharmaceuticals, or consumer goods, AI can be tailored to address your unique quality control challenges. This flexibility allows manufacturers to implement AI gradually and adapt it as their processes evolve.
Customization of AI solutions involves:
a. Industry-specific Algorithms: AI algorithms can be developed or fine-tuned to address industry-specific quality control requirements. For example, the criteria for quality in the automotive industry may differ from those in the food and beverage industry.
b. Integration with Existing Systems: AI systems can be integrated with existing manufacturing and quality control systems, allowing for a seamless transition to AI-driven processes.
c. Scalability: AI solutions can be scaled to accommodate changes in production volume. Whether you’re a small manufacturer or a large industrial operation, AI can be adjusted to meet your needs.
d. User-Friendly Interfaces: User interfaces for AI systems can be designed to be user-friendly, ensuring that operators can easily interact with and interpret the results provided by the AI.
Customizable AI solutions empower manufacturers to take full advantage of AI’s capabilities while aligning with their specific quality control objectives and production environments.
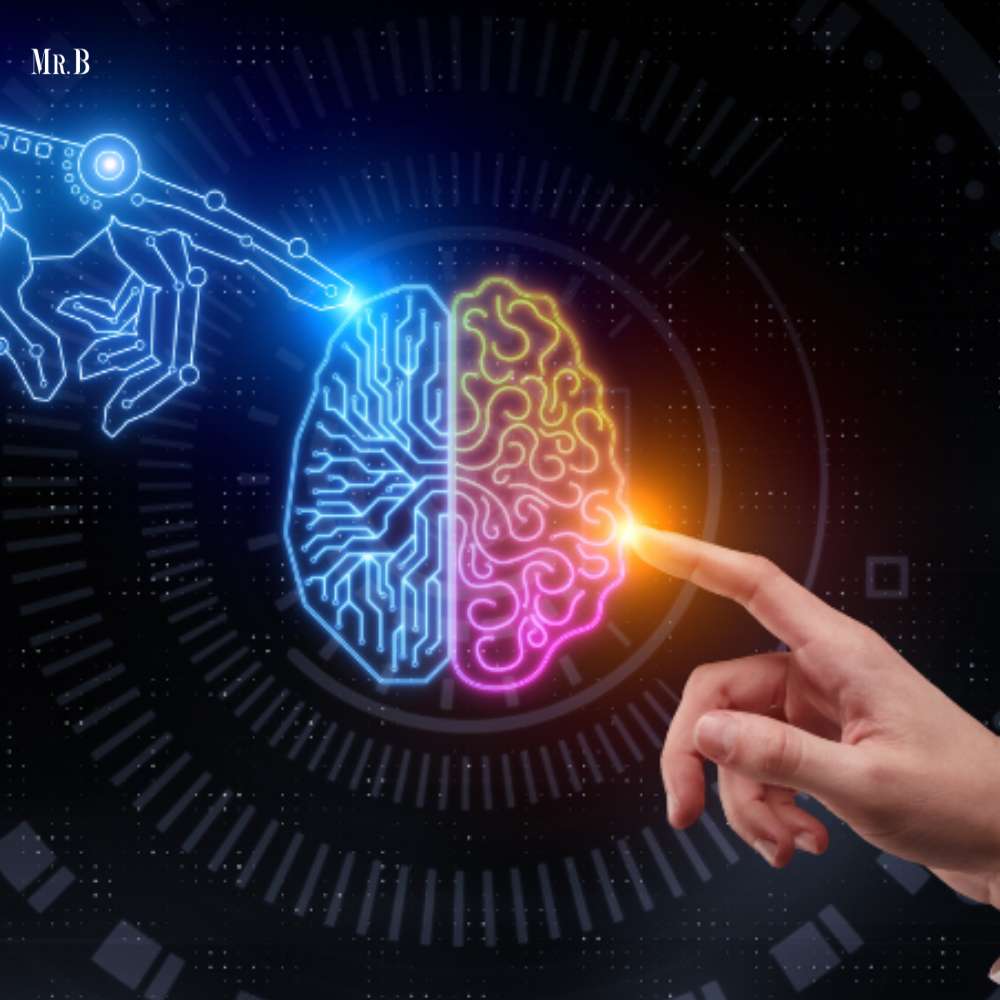
8. Challenges and Considerations
While AI offers significant benefits for quality control in manufacturing, there are challenges and considerations to keep in mind. It’s essential for manufacturers to be aware of these factors as they plan for the implementation of AI-driven quality control solutions:
a. Initial Investment: Implementing AI systems for quality control involves a substantial initial investment. This includes costs for acquiring hardware, software, and AI expertise. Manufacturers should carefully budget for these expenses and consider the long-term benefits of improved product quality and reduced defects.
b. Data Privacy and Security: AI systems rely on vast amounts of data, much of which may be sensitive or proprietary. Manufacturers must take measures to protect the privacy and security of this data, ensuring that it is not vulnerable to breaches or unauthorized access.
c. Workforce Training: The successful implementation of AI, when it comes to quality control in manufacturing requires a trained workforce. Operators and technicians need to understand how to work alongside AI systems, interpret the results they provide, and take appropriate actions based on those results. Adequate training is essential to ensure a smooth transition to AI-driven quality control.
d. Integration Challenges: Integrating AI systems with existing manufacturing processes and systems can be complex. Manufacturers must plan for the integration carefully to avoid disruptions to production.
e. Ethical Considerations: AI-powered quality control raises ethical questions related to decision-making, accountability, and transparency. Manufacturers should establish ethical guidelines and practices to ensure that AI is used responsibly and fairly.
f. Regulatory Compliance: Different industries and regions may have specific regulations and standards related to quality control. Manufacturers must ensure that their AI systems comply with these requirements.
Summing Up
Artificial intelligence has revolutionized quality control in manufacturing. By leveraging machine vision, predictive maintenance, process optimization, real-time monitoring, data analysis, supply chain integration, and customizable solutions, manufacturers can enhance product quality, reduce defects, and improve overall efficiency.
While implementing AI-driven quality control solutions may present challenges, the long-term benefits in terms of cost savings, customer satisfaction, and competitiveness make it a worthwhile investment. As technology continues to advance, AI will play an increasingly integral role in ensuring that products meet the highest standards of quality in the manufacturing industry. Embracing AI is not just a step forward; it’s a leap into the future of quality control.