Organizations rely heavily on data to make informed decisions in the present data-driven world. However, the effectiveness of these decisions is based on the quality of the data being utilized. This is where data quality assessment comes into play. It is a systematic process that helps organizations evaluate the quality of their data and ensure that it meets specific standards and requirements.
In this article, we will explore the importance of data assessment, the criteria used to assess data quality, and best practices for implementing effective data assessments.
What is Data Quality Assessment?
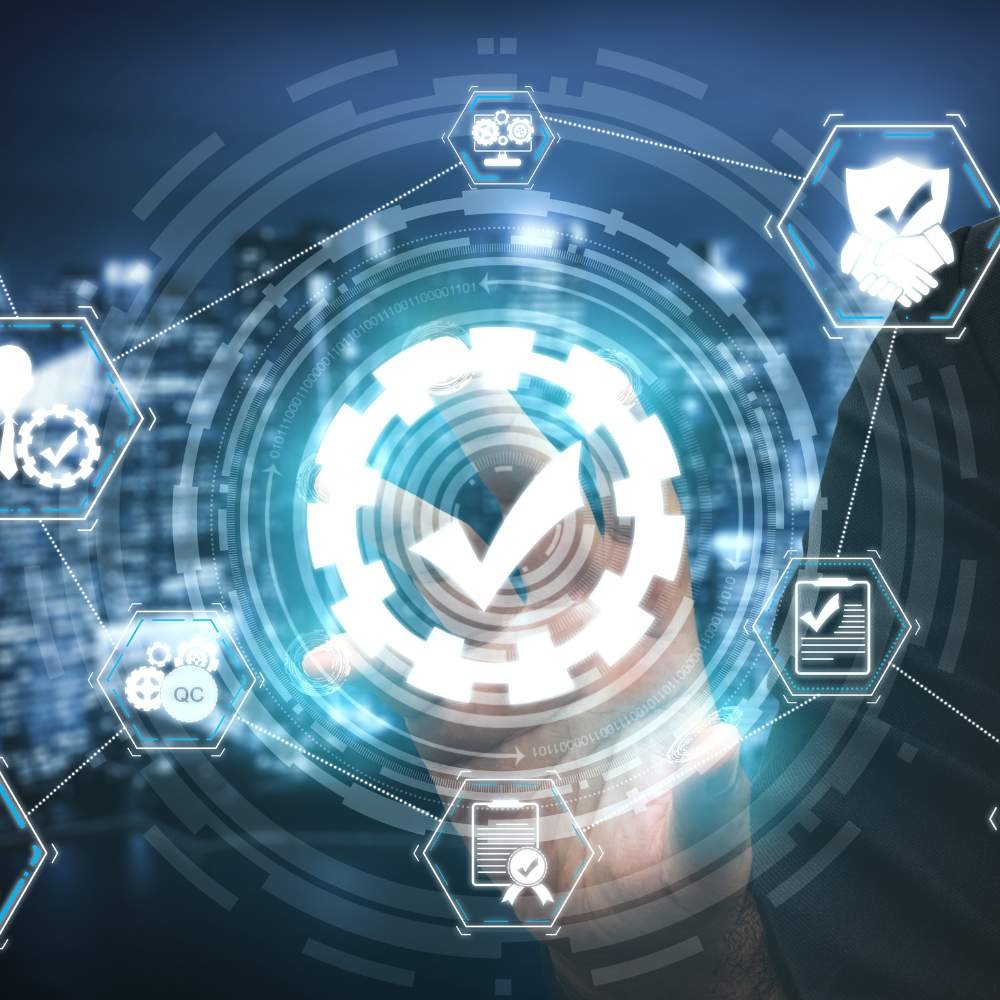
It refers to the process of evaluating the quality of data against defined standards and criteria. This assessment involves checking for accuracy, completeness, consistency, timeliness, and relevance of the data. By conducting a thorough data assessment, organizations can identify issues that may affect their data, leading to more reliable insights and better decision-making.
Why is Data Quality Assessment Important?
- Informed Decision-Making: High-quality data is essential for making informed business decisions. Poor data quality can lead to incorrect conclusions and ultimately affect an organization’s credibility.
- Regulatory Compliance: Many industries are subject to strict regulations concerning data management. A rigid data and quality assessment process helps organizations follow these regulations by ensuring that data is accurate and reliable.
- Operational Efficiency: When organizations assess and improve their data quality, they can ensure processes, reduce errors, and improve operational efficiency. This can lead to significant cost savings and improved productivity.
- Customer Satisfaction: For businesses, maintaining accurate and high-quality customer data is crucial for providing excellent service. A data and quality assessment ensures that customer information is up-to-date and reliable, enhancing the overall customer experience.
Key Features
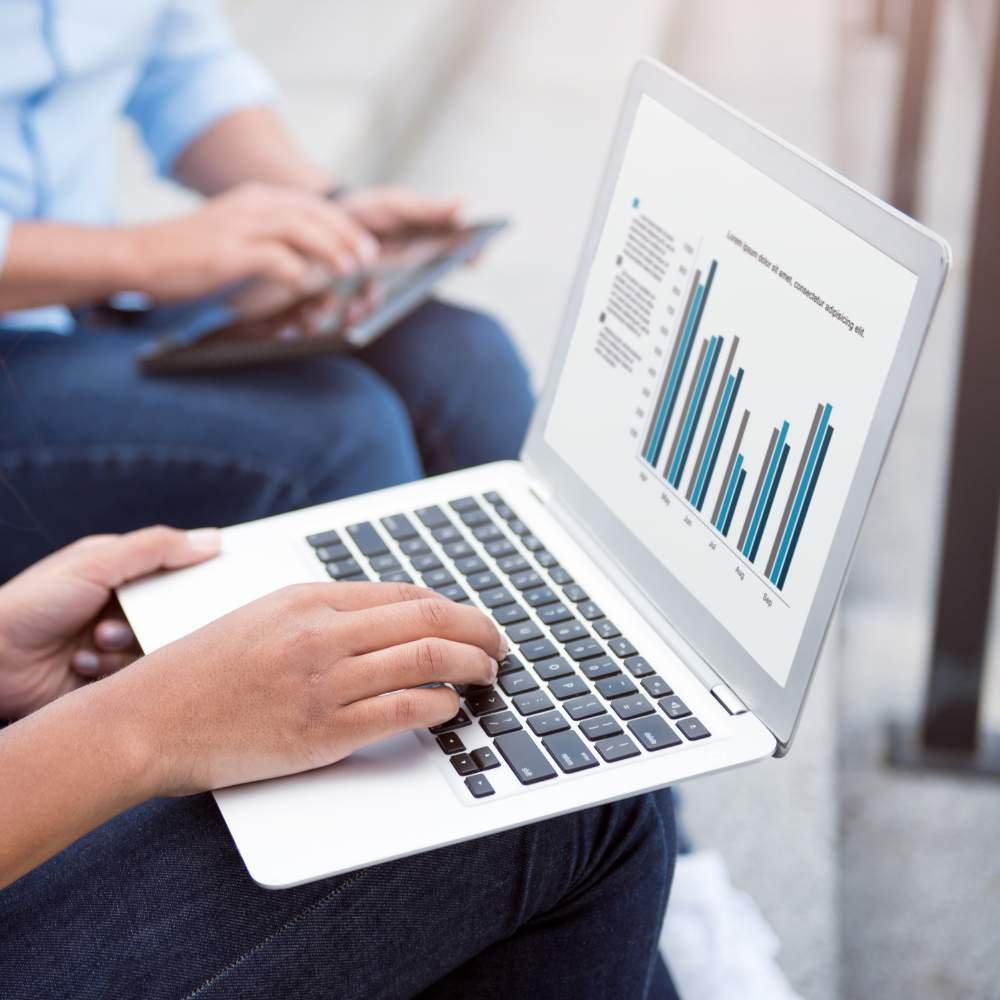
A comprehensive data and quality assessment focuses on several key criteria, each of which ensures the overall quality of the data.
Here are the key features of data quality:
1. Accuracy
Accuracy refers to how well the data represents the values it is supposed to depict. For example, if a database contains customer contact information, accuracy ensures that the details match the actual individuals. During a data assessment, organizations must verify the correctness of data entries through cross-referencing with trusted sources.
2. Completeness
Completeness measures whether all required data is present. Incomplete data can lead to flawed analyses and decision-making. A data quality assessment should identify missing values and ensure that all necessary information is acquired to provide a complete picture.
3. Consistency
Consistency refers to the uniformity of data across different datasets. If the same data is recorded in multiple locations, it should match. A data assessment examines datasets for inconsistencies, ensuring that similar data points do not collide with each other.
4. Timeliness
Timeliness assesses whether data is up-to-date and available when needed. Outdated data can lead to ineffective insights and decisions. A thorough data quality assessment should include checks for the originality of the data, ensuring that it reflects the current state of affairs.
5. Relevance
Relevance evaluates whether the data is applicable to the context in which it is being used. Data that is irrelevant can disrupt the decision-making processes. A well-executed data assessment ensures that only appropriate data is included in analysis and reporting.
Best Practices
To effectively conduct a data quality assessment, organizations should follow these best practices:
1. Define Clear Objectives
Before starting on a data quality assessment, it is crucial to define clear objectives. Identify what you want to achieve with the assessment and which specific data sets will be evaluated. This clarity will guide the assessment process and help you focus on relevant aspects.
2. Utilize Automated Tools
Manual data quality assessment can be time-consuming and prone to errors. Using automated tools can improve the efficiency and accuracy of the assessment process. Many software solutions specialize in data profiling, validation, and cleansing, making it easier to identify issues quickly.
3. Engage Stakeholders
Involve stakeholders from different departments during the assessment process. Their insights can provide valuable context for evaluating data quality and help ensure that the assessment aligns with organizational goals.
4. Establish Data Governance Policies
Implementing strong data governance policies is crucial for maintaining data quality over time. These policies should outline procedures for data entry, management, and regular assessments. A strong governance framework supports ongoing data assessments and promotes accountability.
5. Conduct Regular Assessments
Data quality is not a one-time process. Establish a routine for conducting data quality assessments to monitor data quality over time. Regular assessments help organizations stay on top of data quality issues and ensure that data remains reliable and relevant.
6. Create a Data Quality Dashboard
A data quality dashboard provides a visual representation of data quality metrics. By tracking key performance indicators (KPIs), organizations can quickly identify trends and areas that require improvement. This approach can significantly enhance data quality management efforts.
7. Provide Training and Education
Educating employees about the importance of data quality is essential for promoting a culture of data handling. Provide training on best practices for data entry, maintenance, and assessment. When employees understand the significance of data quality, they are more likely to adapt to standards and contribute to better data management.
Common Challenges
While data quality assessment is critical, organizations may encounter several challenges during the process:
1. Data Silos
Data silos occur when information is stored in isolated systems, making it difficult to access and assess. Breaking down these silos is essential for a data assessment.
2. Lack of Standardization
Inconsistent data formats and definitions can complicate data quality assessments. Establishing standard protocols for data entry and management can help reduce the negative outcomes.
3. Resource Constraints
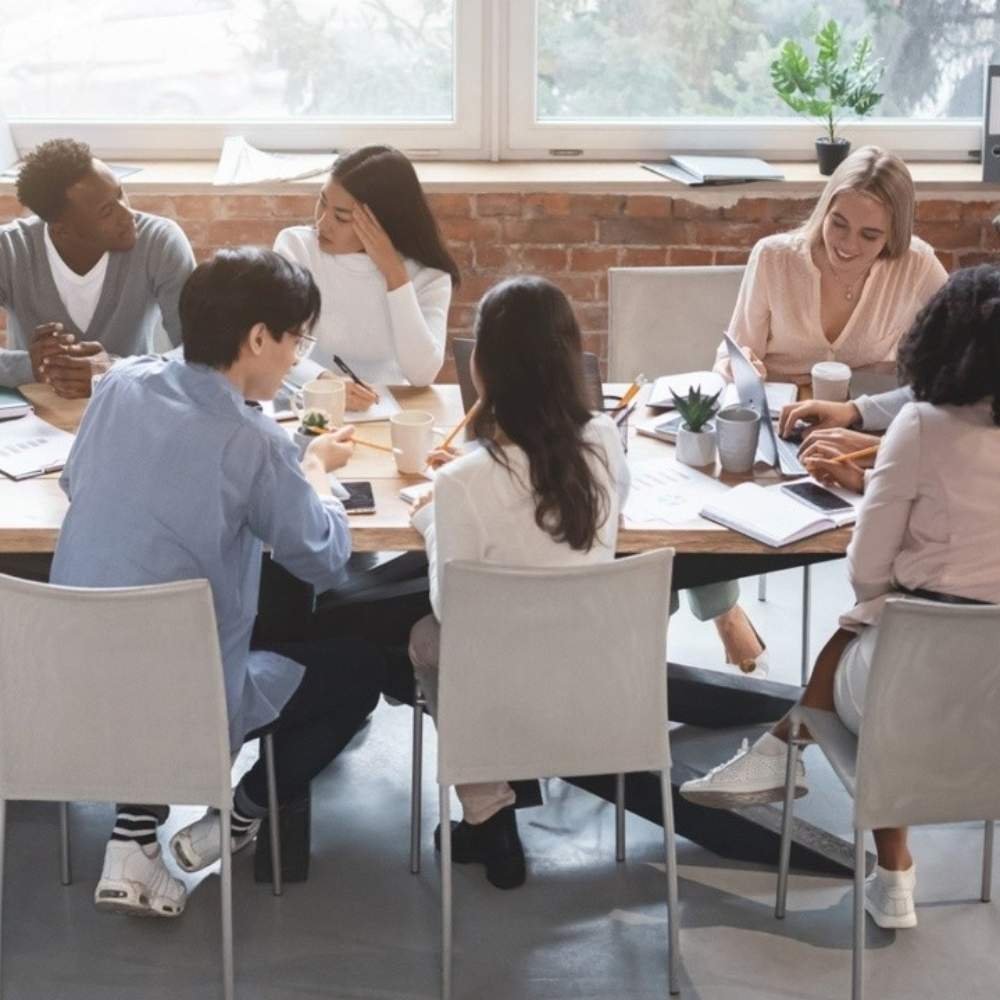
Limited resources, including time and personnel, can alter the effectiveness of a data quality assessment. Organizations should allocate sufficient resources to ensure thorough assessments are conducted.
4. Resistance to Change
Employees may resist changes in data management practices, particularly if they perceive the changes as inefficient. Communicating the benefits of improved data quality can help reduce the resistance and foster a more collaborative environment.
Conclusion
In a world where data drives decision-making, conducting a thorough data quality assessment is essential for organizations to maximize the value of their data. By focusing on accuracy, completeness, consistency, timeliness, and relevance, organizations can ensure that their data is reliable and actionable.
Implementing best practices such as defining clear objectives, utilizing automated tools, engaging stakeholders, and establishing data governance policies will enhance the effectiveness of data and quality assessments. Regular assessments will help organizations stay ahead of data quality issues and ultimately lead to better decision-making, improved operational efficiency, and increased customer satisfaction.
As organizations continue to navigate an increasingly data-centric environment, prioritizing data quality assessment will be crucial for success. By accepting and addressing the challenges associated with data quality, businesses can unlock the full potential of their data and drive meaningful outcomes.